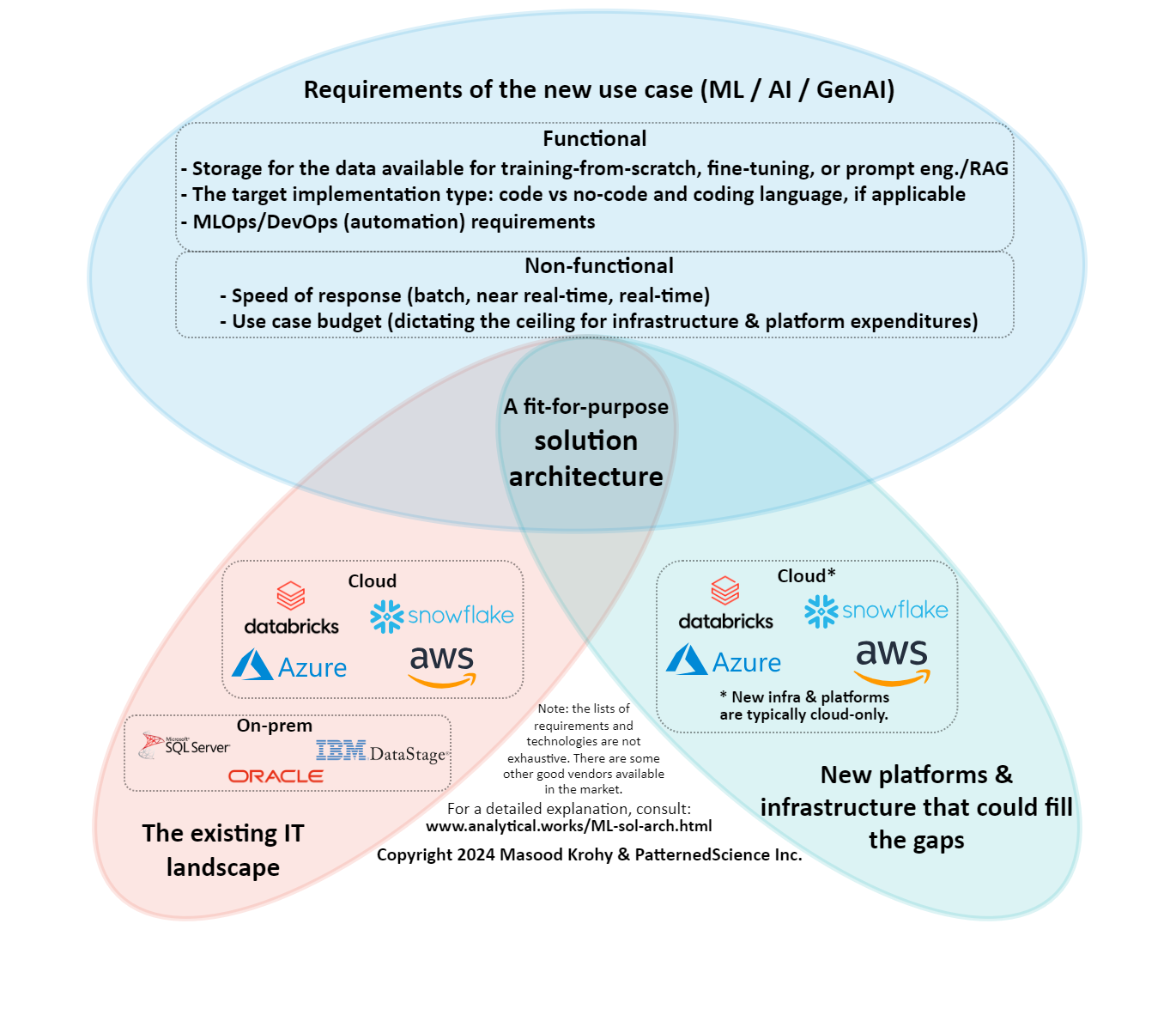
Introduction
Designing a fit-for-purpose solution architecture at an enterprise requires consideration of the following three elements:
- Requirements specific to the new use case (ML / AI / GenAI)
- The existing IT architecture landscape
- New candidate platforms and infrastructure that could help fill the gaps
This short article highlights the importance of identifying the intersection among the three elements so that one ends up with a solution architecture that meets the need of the new use case, uses as much as possible components from the existing IT architecture landscape and fills the gaps with tools, platforms or infrastructure that are needed to be brought in to arrive at a viable, working solution architecture.
Common pitfalls
- Not understanding or documenting enough the functional and non-functional requirements with the help of the business/internal client (typically a Data Scientist / Analyst). While this might be a common issue in all IT projects, properly gathering these requirements often need a deep understanding of data science / ML / AI / GenAI concepts, which is not necessarily available in typical IT departments.
- Not properly taking into account the existing IT landscape. Almost all organisations have legacy systems holding crucial data which need to be brought into the project. Finding the best way to integrate these systems require a broad understanding and experience with various systems by the architect in charge. If the architect has only a narrow experience focused on a specific cloud provider, or a specific platform, the integration needed might not be done in an optimal or sustainable way.
- Not finding the optimal trade-off between reuse of infra/platform already available and acquiring new ones. This is often a delicate balancing act with several factors at play:
a) some internal stakeholders / IT functions might resist any new tool/platform/infra due to inertia and the desire to stay with what they already know best;
b) legitimate desire, primarily on the part of enterprise architects, to limit the number of components in the enterprise IT landscape to have a chance of developing deep expertise and intra-organisational architecture patterns;
c) availability of time and resources for deployment of the new tools/platforms/infrastructure and for the training of the staff to transition properly.
Conclusion
An experienced, independent (vendor-neutral) architect, well-versed in ML / AI / GenAI, is best positioned to work with an organisation to identify, document and communicate the three elements mentioned on top and help an organisation find the intersection between the three. This work can take time and each organisation will have a unique result.